AI Leadership Depends on What is Measured
By:
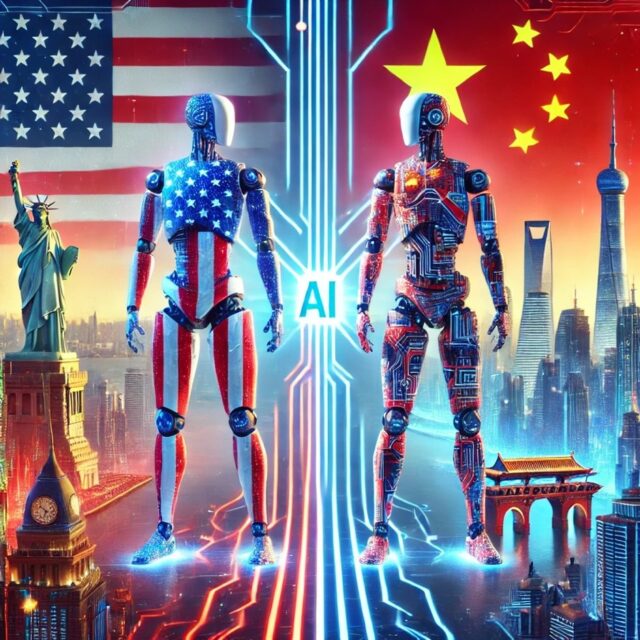
Executive Summary:
- Chinese open-source Large Language Models (LLMs) perform better than Western ones, according to a recent report from an artificial intelligence evaluation organization SuperCLUE.
- Chinese LLMs also excel in cost-efficiency, scalability, and localized applications, with advancements in edge devices and use cases in the health and automotive sectors.
- The benchmark report shows international models still lead in reasoning, multi-modal tasks, and AI agent capabilities.
- Leadership in AI is multi-dimensional and depends on the metrics used for evaluation. Accurate assessment requires contextualizing benchmarks, understanding priorities, and recognizing that distinct national strategies and objectives shape AI development.
In November 2024, the Chinese artificial intelligence (AI) organization SuperCLUE, which describes itself as an “independent, 3rd-party AGI evaluation organization (独立第三方AGI测评机构)” released its “Chinese Large Model Benchmark 2024 October Report” (SuperCLUE, November 8; WeChat/SuperCLUE, November 18). The report offers insights into the global state of large language models (LLMs) and captures trends in the current AI landscape. It uses the SuperCLUE framework to assess the progress and challenges faced by models from the People’s Republic of China (PRC) and international leaders.
The report argues that the PRC’s AI ecosystem has made significant strides since May 2023, narrowing performance gaps with top Western models. However, the release of OpenAI’s o1-preview widened the capability gap to 8.19 percent in August 2024, highlighting persistent challenges for PRC models in catching up on advanced reasoning and multimodal capabilities. Some of the report’s conclusions, such as claims that “domestic open-source models are leading globally,” indicate the risks of attempting to measure AI “leadership.” LLMs built in the PRC tend to exhibit different strengths than those in the United States, and different skillsets may have more value in one country than in the other.
SuperCLUE Framework Shows Chinese Models’ Progress
Evaluating AI models is crucial for benchmarking LLMs’ progress and identifying areas for improvement. The Chinese Language Understanding Evaluation (CLUE) framework, established in 2019, serves as a neutral benchmark widely used in academic and industrial contexts. It includes specialized variants like FewCLUE for few-shot learning and KgCLUE for knowledge graph integration. [1] The SuperCLUE framework is a gold standard benchmark. Building on CLUE, it evaluates models across academic, industrial, and real-world user scenarios based on a range of metrics that include hard tasks, multi-modal integration, and real-time adaptability. The benchmark assigns a score for models’ performance on a range of tasks before calculating an overall score out of 100 for each model. Models that score above 60 are described as exceeding the standard performance level. This is because most models achieve scores in the range of 55–60 on tasks, so a threshold of 60 allows for a clear differentiation between models that perform at or above the average level and those that fall below it. [2]
SuperCLUE evaluations reveal models’ strengths and areas for improvement, particularly in STEM and humanities-related tasks. In STEM tasks, international models like o1-preview lead with a SuperCLUE score of 86.07 out of 100. PRC models, including Qwen2.5-72B-Instruct and 360gpt2-pro, have narrowed the gap to the point of being competitive contenders in technical and analytical domains. In humanities and knowledge-based tasks, the gap is even narrower. For instance, in tasks like historical analysis and contextual role-playing, ChatGPT-4o-latest scores 77.10, with leading PRC models trailing at 76.96, indicating the latter’s capabilities in language-intensive applications.
Hard tasks and reasoning abilities assess LLMs’ abilities to perform multi-step logical reasoning, follow detailed instructions, and synthesize complex information. International models like o1-preview outperform PRC counterparts such as GLM-4-Plus in such tasks. For instance, in one test detailed in the report, the researchers asked models to assist students with selecting courses by balancing student outcomes while adhering to budget constraints and solving for variables such as course numbers, class durations, and student counts. While o1-preview provided detailed reasoning and accurate solutions, PRC models often missed crucial steps. The same was true for tasks requiring models to follow instructions, like following formatting rules when generating responses.
Multi-modal models integrate and process information from text, images, and other media. This is essential for real-world applications like image recognition, multi-modal storytelling, and cross-medium reasoning. International models like GPT-4o maintain a significant edge in multi-modal tasks. For instance, GPT-4o successfully generated narratives for a video advertisement. PRC models, including hunyuan-vision and SenseChat-Vision 5.5, have advanced in performing culturally specific tasks. For instance, SenseChat-Vision 5.5 accurately described intricate patterns in traditional Chinese embroidery. However, these models still face challenges achieving broader adaptability across diverse multi-modal tasks.
Chinese Models Focus on Smaller Models, Industrial Applications
PRC models appear to have an edge among small-scale models with 10 billion and 5 billion parameters. [3] Such models are intended for use in resource-constrained environments such as smartphones, IoT devices, and robotics. Among models with 10 billion parameters, Qwen2.5-7B-Instruct is the best of those surveyed, with 60.61 points, surpassing the 60-point benchmark, followed by GLM-4-9B-Chat at 56.83. Internationally, Gemma-2-9b-it achieves 55.48. In the 5B category, MiniCPM3-4B tops the rankings at 53.16, excelling in STEM (63.04) and humanities (69.87) tasks and outperforming comparable models like Phi-3-Mini-4K-Instruct. Overall, six models under the 10 billion parameters threshold scored above 50, with four being domestic. Notably, PRC models averaged 7.14 points higher than their international counterparts in this category. By balancing performance, efficiency, and cost, these smaller models are well-positioned to drive AI adoption in a range of localized applications.
Code generation and AI agent capabilities are benchmarks for assessing the utility of LLMs for tasks such as computer programming and performing autonomous operations in multi-step workflows. Currently, international models like o1-preview lead significantly in code generation, with many PRC models producing incomplete or inconsistent outputs by comparison. Similarly, as AI agents, GPT-4 outperforms other models in task decomposition, API integration (using an interface to connect different software systems), and autonomous decision-making. PRC models have progressed in API usage, but still lag in their ability to understand nuanced reasoning.
PRC models have excelled in industry-specific applications, particularly in the automotive, health, and finance sectors. In the automotive sector, intelligent cockpit systems powered by these models perform well in voice recognition, route optimization, and user interface customization. In healthcare, they outperform their international counterparts in tasks like diagnostic assistance and medical report generation. Some slight gaps remain, though closing them will require broader datasets and deeper industry collaboration. By focusing on tailored use cases, PRC models have carved out a competitive niche, which they will likely attempt to consolidate into global leadership.
Pitfalls in Measuring ‘AI Leadership’
The AI landscape is in flux, limiting the ability to draw robust conclusions about the state of the field. Much attention centers on the race for frontier models with massive compute capabilities and token processing power. However, this only constitutes one part of the picture. The PRC’s AI priorities often diverge—something that must be considered in any assessment of global AI progress.
The approach to AI development taken in the PRC has focused on cost-efficiency and resource optimization, contrasting with the maximalist approaches seen in the West. Technology entrepreneur Kai-Fu Lee recently claimed that his company, 01.AI, had achieved impressive results using only 2,000 GPUs—less than 2 percent of the resources typically used by OpenAI. With just over $3 million spent on pre-training, 01.AI achieved performance surpassing GPT-4 at a fraction of the cost. This focus extends to inference, where costs dropped from $1.40 per million tokens in June 2023 to $0.10 by September and were projected to reach $0.03 by mid-2024 (YouTube/Peter H. Diamandis, December 5). Scalable, cost-effective AI solutions that can diffuse across industries may be seen as having higher utility than focusing on achieving advanced general intelligence (AGI). This constitutes a divergent school of thought in the mainstream PRC approach to building LLMs compared to that of the West, which suggests different visions for AI’s role in society.
This divergence makes comparisons fraught. Chinese-language models excel in benchmarks tailored to highlight their strengths, but gaps persist in areas like hard reasoning and advanced logic, where Western models consistently are dominant even within Chinese-language scenarios. The PRC offers a strong case for measuring leadership by the extent of integration into scalable, everyday applications.
Conclusion
Neither the PRC nor the United States is likely to achieve absolute dominance in AI at this stage. Evaluating AI “leadership” depends on the measurement criteria, which may reflect a degree of selection bias due to differences in what is deemed of value. Currently, PRC models are narrowing the gap in general language capabilities but lag in advanced reasoning, AI agent functionality, and multimodal applications. Conversely, the PRC excels in small models and edge AI (deploying AI models directly to devices), prioritizing scalability and industrial applications.
The “Chinese Large Model Benchmark 2024 October Report” is valuable, but it emphasizes linguistic and general capabilities while overlooking adaptability, creativity, and cross-cultural functionality. Accurate assessments require contextualizing results, critically evaluating metrics, and understanding these differing strategies to form a balanced view of global AI progress.
Notes
[1] Few-shot learning is a machine learning approach where a model is trained to learn and generalize from a very limited number of examples. It is typically used to train models for classification tasks when suitable training data is scarce (IBM, accessed December 13). Knowledge graph integration refers to the process of incorporating information from structured data representations, called knowledge graphs (KGs), into machine learning models or applications. The process organizes data in a way that mirrors real-world connections, allowing machines to reason about facts and relationships (IBM, accessed December 13)
[2] Liang Xu et al. “SuperCLUE: A Comprehensive Chinese Large Language Model Benchmark.” arXiv preprint arXiv:2307.15020 (2023).
[3] LLM parameters are like adjustable dials that control how a model responds. They define the behavior of the model. For comparison, ChatGPT-4 has approximately 1.8 trillion parameters.